This is the second article in a four-part series about applying Department of Energy big data and supercomputing expertise to cancer research.
Visionary science fiction writers like Arthur C. Clarke often pictured a world in which humans and computers communicated using natural spoken language. But that vision had yet to materialize in 2001, much less now.
Creating computers that can understand human language and make intelligent decisions based on programmed reasoning skills is a more challenging computational problem than most people appreciate, says Georgia D. (Gina) Tourassi, director of the Health Data Sciences Institute (HDSI) at Oak Ridge National Laboratory and co-principal investigator of the Joint Design of Advanced Computing Solutions for Cancer collaboration between DOE and the National Cancer Institute (NCI).
Yet, cancer experts agree, having the computational tools to automatically collect key data from medical records could be a gold mine for researchers seeking to rapidly assess what’s working and what’s not in cancer care.
When a cancer patient visits an oncologist, fills a prescription or gets a lab test, a record is generated. But capturing such day-to-day cancer treatment data has proven elusive. Most is recorded by doctors as free-text narratives. And doctors have their own, slightly different shorthand.
The research team is developing large-scale computational tools to allow predictive modeling for individual patients.
Natural-language data-processing requires complex algorithms combined with machine learning for deep comprehension, a distilling of important contextual information from unstructured text. For instance, physician terminology often can have multiple meanings depending on the context. Ideally, Tourassi says, you want the computer to do all the heavy lifting automatically.
“There are a lot of intermediate data in terms of recurrence, metastases, treatment response that are not currently captured in the cancer surveillance data,” Tourassi says. “Having people capture this data manually from electronic health records is not scalable. We need to monitor more people for longer periods, and that’s too much data for human curators. In addition, there is the problem that data collection is done across different clinical sites because people get care in several places.”
Tourassi is directing a pilot project that will integrate cancer data with large-scale computing as part of the President’s Precision Medicine Initiative. This Oak Ridge-centered project starts with data collected nationally through the NCI’s Surveillance, Epidemiology and End Results (SEER) Program from population-based cancer registries.
Tourassi’s 20-member research team is developing natural language processing and machine-learning tools for deep text comprehension of unstructured clinical text, such as pathology and radiology clinical reports. The goal, she says, is to track cancer incidence over time and identify patterns for various populations. The pilot project is working with data already collected at four state cancer registries: Kentucky, Louisiana, Georgia and Washington. Eventually, the SEER data will be connected with other data sources to let researchers examine things such as cost and quality of care.
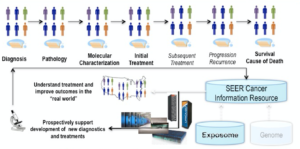
A schematic (click to enlarge) of how cancer surveillance data will be captured from an entire patient population, with molecular information fed through the National Cancer Institute’s SEER data base. Image courtesy of Georgia Tourassi, Oak Ridge National Laboratory.
“The cancer surveillance program is almost a reality check on how we are doing in the war on cancer,” Tourassi says. The goal: to help capture information that’s vital for diagnosing and treating cancer in today’s complex medical and social environment. “We also want to enhance the existing system by expanding the breadth of data captured to understand the broad drivers of cancer outcomes: social, psychological and ecological variables, not only what kind of treatments people receive but how the healthcare delivery system plays a role.”
Besides developing natural-language tools, the team is developing scalable visual analytics for cancer surveillance data, including the effects of environmental exposures – the so-called exposome – on populations.
Finally, in the pilot’s most ambitious leg, the research team is developing large-scale computational tools to allow predictive modeling for individual patients.
The purpose “is to enable in silico, large-scale recommendation of precision cancer therapies and prediction of their expected impact in the real world,” Tourassi says. “We need to become prescriptive and let data-driven modeling and simulation guide us into what intervention must be delivered to which patient at which time point for maximum long-term benefit. In order to do that we have to be able to model the whole system. Modeling and simulation of complex systems and processes at scale is something that the Department of Energy has been doing across a number of science domains, particularly the regulation and operation of nuclear power plants.”
The challenge in working with human life is that biological systems are not completely mechanistic. That’s why data-driven approaches and machine learning must be built into the scientific discovery process in our fight against cancer, Tourassi says. The next generation of grand challenges is both computation- and data-intensive. And exascale computing, with machines about a thousand times more powerful than today’s supercomputers, will pave the way.
Part of the DOE-NCI collaboration involves adapting tools from academia so they scale to supercomputers, identifying gaps and developing new machine-learning tools for algorithmic development to support the project. That’s where the $2.5 million budget for scalable deep learning called the CANcer Distributed Learning Environment (CANDLE) comes in. Tourassi and her team will develop scalable deep neural networks, designed to port to eventual exascale computers, to demonstrate automated capture and accurate extraction of cancer surveillance information at the population level.
Beyond cancer, the infrastructure will inform future computer architectures and make high-performance computing more amenable to solving biomedical research questions that assemble big data, machine learning, and modeling and simulation.
“We are using high-performance computing to bridge the gap between precision medicine and population health,” Tourassi says.